Choosing the best storage solution in GCP
Barbora Cernakova11 min read
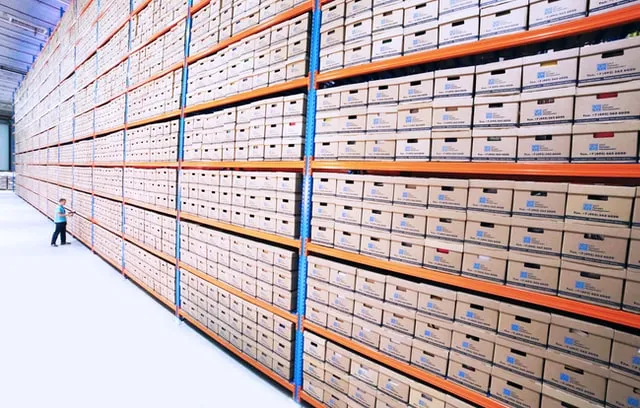
Are you new to GCP, looking to make sense of all the storage offerings GCP provides or simply hoping to learn something new? You’re in the right place!
In this blog we’re going to compare the key differences between GCP’s numerous storage solutions. We will start by taking a look at a handy decision tree which gives you an overview of the various options. We will then categorise the available storage solutions into blob, relational, NoSQL, file, block and other storage. For each option, we will delve a bit deeper into the specifics and we will highlight the best suited use cases as well as flag any potential downsides. Let’s get started!
Decision tree
As promised, we’re starting with a decision tree to help you choose the best storage tool for your use case. Don’t worry if you’re not sure what all this means - we’ll take a look at each tool below.
Now let’s look at each of the options listed above in a bit more detail.
Blob storage
Blob storage allows you to store unstructured object data. This means that we’re looking at data which often consists of items (e.g. media files) which don’t need to sit within a file structure.
Cloud Storage
We’re starting with one of the most commonly used storage options - Cloud Storage. Cloud Storage is GCP’s blob storage solution and it is often used alongside other tools such as Compute Engine (service for creating and running virtual machines), App Engine (platform for building highly scalable applications using serverless) and BigQuery (data warehousing solution - more info below). In terms of its structure, it uses buckets to group items.
Benefits
- Buckets are really powerful organisation tools, which allow you to control lots of properties, including lifecycle policies (e.g. delete object 2 years after creation) and object versioning (by default, object versioning is switched off, so if updated, the object will be overwritten).
- You can choose to either host your bucket in a single region, or it can be dual-regional or multi-regional. The dual-regional option in particular isn’t seen across many storage options. It can be a great way of saving on costs (in comparison to multi-regional buckets) whilst bringing your data closer to your users and making sure it is available even in the event of a regional outage.
- You can choose a suitable storage class based on how often you need to access your data. The four storage classes available are standard (access regularly), nearline (access once a month), coldline (access once a year) and archive (access less often than once a year), with the cost of storage decreasing with each subsequent class.
Downsides
- Cloud Storage cannot mimic file structure of nested folders. Each item is accessed directly via its own url.
- Coldline and archive classes carry an associated retrieval cost, which will be charged when you access your data. This means that the costs can sneak up on you, especially if you’re not keeping track of how often you’re accessing objects in your long-term storage buckets.
In terms of common use cases, Cloud Storage is an excellent option for archiving data for audits and for storing data for disaster recovery. It is also great for minimising latency for users who download large data objects since you can choose dual-regional or multi-regional storage to bring your data closer to your users.
Relational storage
Unlike with blob storage, relational databases organise data into tables which can be linked to each other. They are the industry standard and GCP provides a couple of options for storing relational data within the cloud.
Cloud SQL
Cloud SQL is the obvious choice when setting up a relational database in GCP. It provides a fully managed service which now allows you to create instances of MySQL, PostgreSQL as well as Microsoft SQL Server.
Benefits
- Easy to migrate to from on-premise relational databases, either using MySQL dump or CSV file imports.
- Variety of methods for connecting to your database, including access via private IP and Cloud SQL proxy.
- Automatic data replication with failover.
- Automated storage capacity management.
Downsides
- Storage capacity is limited to 30 TB, which may catch some users out.
- Cloud SQL is great for vertical scaling, but if you’re interested in horizontal scalability, you’d best look elsewhere.
Cloud SQL is an excellent option if you’re looking to replicate your on-premise database within the cloud, or if you’d simply like to stick with a database you know already. This is exactly what happened with AutoTrader, a digital automotive marketplace. They were looking to move their on-premise infrastructure to the cloud, and they chose to move their existing Oracle database into Cloud SQL.
Cloud Spanner
GCP’s alternative to Cloud SQL is Cloud Spanner. Cloud Spanner provides a relational database structure with the added advantage of horizontal scaling.
Benefits
- If you’re concerned that Cloud SQL doesn’t provide enough capacity for your needs, Cloud Spanner is definitely worth a consideration since it scales to PB capacity.
- Support for Google Standard SQL as well as PostgreSQL.
- It provides strong consistency, meaning that once a user makes a change to the data, the update will be consistent for all users immediately.
- It automatically replicates data across multiple zones.
Downsides
- Whilst you can create backups on demand, there is no in-built option to generate them automatically.
- Cloud Spanner isn’t MySQL compliant.
Since Cloud Spanner offers strong consistency globally, it is well suited to financial applications. A fun real-life example is Pokemon Go, which uses Cloud Spanner due to the aforementioned feature.
NoSQL storage
By comparison to relational storage, NoSQL databases store data in non-tabular formats. This can include document storage, key-value pairs or wide-column databases. GCP offers two main NoSQL databases, which we will explore below.
Cloud Bigtable
Cloud Bigtable consists of sparsely populated columns, where rows are indexed by a single key. It is possible to group columns into column families to capture which columns are related. Each row-column intersection can contain multiple cells at different timestamps, and this allows Cloud Bigtable to record a history of data updates.
Benefits
- It can comfortably handle PB capacity.
- It offers very low latency, consistently hitting targets of sub-10ms.
- Easy integration with big data tools, including Hadoop and BigQuery.
- Clusters can be resized with no downtime.
Downsides
- The smallest possible cluster consists of 3 nodes and provides 30,000 operations per second.
- Cloud Bigtable doesn’t scale to zero, so you pay for nodes regardless of whether they are being used.
Cloud Bigtable works well for operational and analytical use cases, and is often used with IoT applications. A real-life example is Dow Jones, a news content and business information provider, who uses Cloud Bigtable to store their data before processing it for analytics.
Firestore (previously Datastore)
Previously known as Datastore, Firestore is the new generation of this NoSQL document database. Unlike Cloud Bigtable, it uses JSON to store data.
Benefits
- Integration with Firebase - GCP’s app development offering.
- Firestore offers strong consistency. This is a typical feature of relational databases.
- Multi-regional replication is available.
- It provides ACID transactions, allowing you to be confident that there are no transactional discrepancies within your data.
Downsides
- It only scales up to TB capacity.
Firestore is an excellent choice for mobile and web applications at global scale. Thanks to its integration with Firebase, setting up Firestore can be a seamless experience. An example of Firestore being used in the real life is The New York Times, who use Firestore to support their real-time rich text editing tool.
File and block storage
We are now moving on from nonSQL storage to file storage, by which we mean storage which allows us to replicate the commonly used file structure of nested folders. The best way of thinking about it is that it mimics the file system of a computer. Block storage, by comparison, stores data as separate pieces, where each piece can be uniquely identified. Block storage is very performant since it allows for data to be accessed via multiple paths.
Filestore
Filestore (not to be confused with Firestore) is GCP’s fully managed network attached storage (NAS) option. It is often used alongside instances of Compute Engine and Kubernetes Engine. Filestore also offers four tiers (basic HDD, basic SSD, high scale SSD and enterprise) to allow you to pick the best solution for your needs.
Benefits
- Filestore offers high performance - throughput of 25 GB/s and 920,000 operations per second.
- It scales up to 100 TB capacity.
- Capacity automatically scales up and down to minimise cost.
Downsides
- It is rarely used without an associated Compute Engine or Kubernetes Engine instance.
Filestore is often used for file sharing as well as high performance computing, e.g. genomics processing.
Persistent disk
We are now delving into the world of storage solutions for Compute Engine. GCP provides a variety of options within the persistent disk offering. As expected, at the the highest level of categorisation, you can choose between SSD and HDD.
Benefits
- Automatic encryption using GCP-supplied keys or your own.
- Durable storage - there is very low risk of loss of data, with the risk lower for regional persistent disks.
- There is no charge for operations.
- The disk types include standard, balanced, extreme or SSD, so you have a lot of freedom to choose the best suited alternative.
Downsides
- Its usage is primarily restricted to Compute Engine.
- A persistent disk can also be used with Kubernetes Engine as storage for persistent volumes.
- Persistent disks can be zonal or regional, so they aren’t well suited for global applications.
The most common use case for persistent disks is, unsurprisingly, attaching them to Compute Engine instances.
Local ephemeral disk
The final file storage type we’ll cover is local ephemeral disk. The main difference between local ephemeral disks and persistent disks hides in the name - persistent disks retain data even if they are not attached to a virtual machine and the data will be kept until the disk is deleted. By comparison, the data stored on local disks is lost as soon as the virtual machine it is attached to is stopped or deleted.
Benefits
- Local disks provide higher throughput and lower latency than persistent disks since they are physically attached to the server.
- It is possible to attach up to 9 TB per instance using a maximum of 24 local disks.
Downsides
- Local disks are only available as SSD, so HDD local disks don’t exist.
- You can’t attach local disks to certain virtual machine types.
- Whilst you can be confident that your disk is always encrypted using GCP-supplied keys, you can’t supply your own encryption keys.
Local disks are best suited if you need fast scratch disk or cache.
Other storage types
In this final section we introduce the remaining two storage solutions which don’t fit well into any of the categories mentioned above.
Memorystore
Memorystore is GCP’s fully managed service for Redis and Memcached. If you are interested in using either Redis or Memcached but don’t want the hassle of managing them, Memorystore is the storage option for you.
Benefits
- Memorystore provides sub millisecond latency for lightning fast data access.
- It is easy to migrate from an existing Redis or Memcached instance using the lift-and-shift functionality.
- Memorystore natively integrates with GCP’s IAM service for robust security.
Downsides
- Memorystore’s capacity is limited to 300 GB.
- If cost is a significant factor, then you should consider hosting your own Redis or Memcached instance.
BigQuery
GCP’s data warehouse solution, BigQuery can handle data storage as well as analysis. It isn’t the most common storage solution, and is often used alongside other storage options for data analysis only.
Benefits
- BigQuery is great for data analysis - all your data can be in one place so you don’t have to worry about data ingestion.
- It provides many specialised services, including BigQuery ML for machine learning and BigQuery BI Engine for interactive data analysis.
- You can also stream data directly into BigQuery from other storage solutions.
Downsides
- Cost is relatively high, so it may not be the most efficient long-term solution.
- It’s not recommended to use BigQuery for data storage unless you want to use its analysis capabilities as well.
One of GCP’s customers using BigQuery is The Home Depot, who use this tool to keep track of their stock across 50,000+ items and 2,000+ locations.
Conclusion
GCP provides a massive variety of data storage tools for all sorts of use cases. Selecting the best option can be difficult, since there are so many tools to choose from. My best advice is to start from your data type, this will often significantly narrow down the available possibilities. Afterwards, considering the location of your data (e.g. zonal or global), required consistency and capacity will help you decide. Make sure to consider cost as well - you can use GCP’s pricing calculator to get a sense of how expensive different solutions would be.
I hope that you’re this leaving this blog post with a clearer idea of the different storage options GCP provides. Remember that if none of the tools listed here fits your use case, you can always build your own solution on Compute Engine. Best of luck with your cloud journey!